Urgency
This page describes urgency, the key metric used to help fact checkers prioritise their work
Overview
The goal of fact checking is to find and debunk net new misleading and deceptive content. There is little point in fact checking information or claims that have been fact-checked already, as this would be duplicating work. Similarly, there is little point in spending effort fact checking claims that are weeks old, as this effort will have little impact. Finally, time is always wasted discovering fact checkable information. Content may not include claims, or claims made may be of little harm.
To counter these issues, we have introduced the concept of Urgency Scoring, designed to maximise the rate of finding net new misleading and deceptive content.
The urgency score
Urgency is the scoring mechanism used in Logically Accelerate to indicate the need to fact check a particular piece of content, relative to other content. Urgency is specific to the topic at hand, as no two topics are alike. Urgency allows the fact checker to prioritise their work, in order to maximise the rate of finding net new misinformation.
Urgency is modelled on the real needs and workflow of fact checkers when they consider what information to fact check. To this end, it tries to model the pain points discussed above - lack of novelty or duplication of work, recency of the claims and the relevance of the video for fact checking. Simply put, we model urgency in the following way:
Urgency = Relevance + Novelty + Recency
A piece of content which is more urgent relative to an other, would be one which has scored higher in a combination of how novel the claims inside the video are judged to be, how recently the information has been posted or uploaded and how relevant the video is in terms of the potential to contain harmful fact checkable information.
Relevance
Relevance is a score which captures the potential of a piece of content to contain harmful fact checkable claims. We break this down into two categories - firstly the potential to contain fact checkable claims and secondly, the potential of containing harmful misinformation.
Relevance = Claims Detected + Harm
Claim Detection
The number of claims detected within a piece of content by our AI directly impacts the potential of the content containing information that can be fact checked. Claims are defined to be factual assertions made within the content that can be empirically verified or debunked. This includes, but is not limited to:
- Concrete factual assertions that can be tested, confirmed, or disproven.
- Statistics or numerical data that can be checked against reliable sources.
- Scientific, academic or expert-related information/concepts that can be checked against reliable sources.
NB: Our current score is calibrated to focus on newsworthy and political events.
When detecting claims, we are not making a judgement about the truthfulness of the claim.
The value of detecting claims can vary with each topic the user is interested in, and therefore we allow the user to modify the weighting of the detected claims in the final score on a per-topic basis.
Harm
Harm is a difficult concept to measure directly, so we use a number of proxy metrics. Firstly, we use the amount of toxic information detected within the content we are analysing, with a high toxicity score indicating a higher level of potential harm.
Secondly, we use the content engagement metrics such as likes, shares, views, comments, which are not a direct measure of harm, but rather a measure of the potential reach of any harm caused if the content did contain misinformation.
Novelty
The novelty of a claim when considering its contribution to net new misinformation is directly related to the amount of existing fact checks that are strongly related to the claim, and that have been checked by other credible fact checkers.
That is to say, a claim which has no existing fact checks will be highly novel, and a claim with many related fact checks is likely to be quite common.
In order to measure novelty, we therefore look at the number of related claims for each claim detected within a piece of content. An additional signal on fact check matching is the source of the check. Claims that have been previously fact checked by IFCN verified signatories can be given a higher weight compared to non IFCN fact checkers who may have published less credible fact checks.
We use Factiverse for claim matching, as well as Fact Checks provided by Logically Facts.
Recency
The recency of a piece of content is directly related to its post or upload date. We have a devised score that directly reflects this:
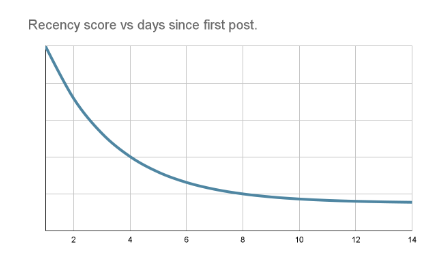
By adjusting the weighting on the recency score, you can decide how important it is the cent you prioritize as urgency was posted recently or not.
Updated 7 months ago